

Feature engineering is done using pre-trained GloVe word embeddings to extract English semantic context vector, hand-crafted features using subjective lexicon Hindi-SentiWordNet to generate the SentiHindi feature vector and an auxiliary pragmatic feature vector depicting the count of pragmatic markers in tweet. The randomly sampled dataset contains 3000 sarcastic and 3000 non-sarcastic bilingual Hinglish (Hindi +English) tweets. To evaluate the performance of the proposed model, real-time mash-up tweets are extracted on the trending political (#government) and entertainment (#cricket, #bollywood) posts on Twitter.

The proposed model is a hybrid of bidirectional long short-term memory with a softmax attention layer and convolution neural network for real-time sarcasm detection.

This research proposes sarcasm detection using deep learning in code-switch tweets, specifically the mash-up of English with Indian native language, Hindi. As we will see, the plain machine learning algorithm applications are just as or in many cases are much better in performance than the more analytical approach.Īnalyzing explicit and clear sentiment is challenging owing to the growing use of emblematic and multilingual language constructs. The designed system is an effort which classifies Hindi as well as Marathi text transliterated (Romanized) documents automatically using supervised learning methods (KNN), Naïve Bayes and Support Vector Machine (SVM)) and ontology based classification and results are compared to in order to decide which methodology is better suited in handling of these documents. The work already performed in this area is progressing at great pace and this work hopes to be a way to push that work further. A lot of these texts are available in transliterated code-mixed form, which due to the complexity are very difficult to analyze.
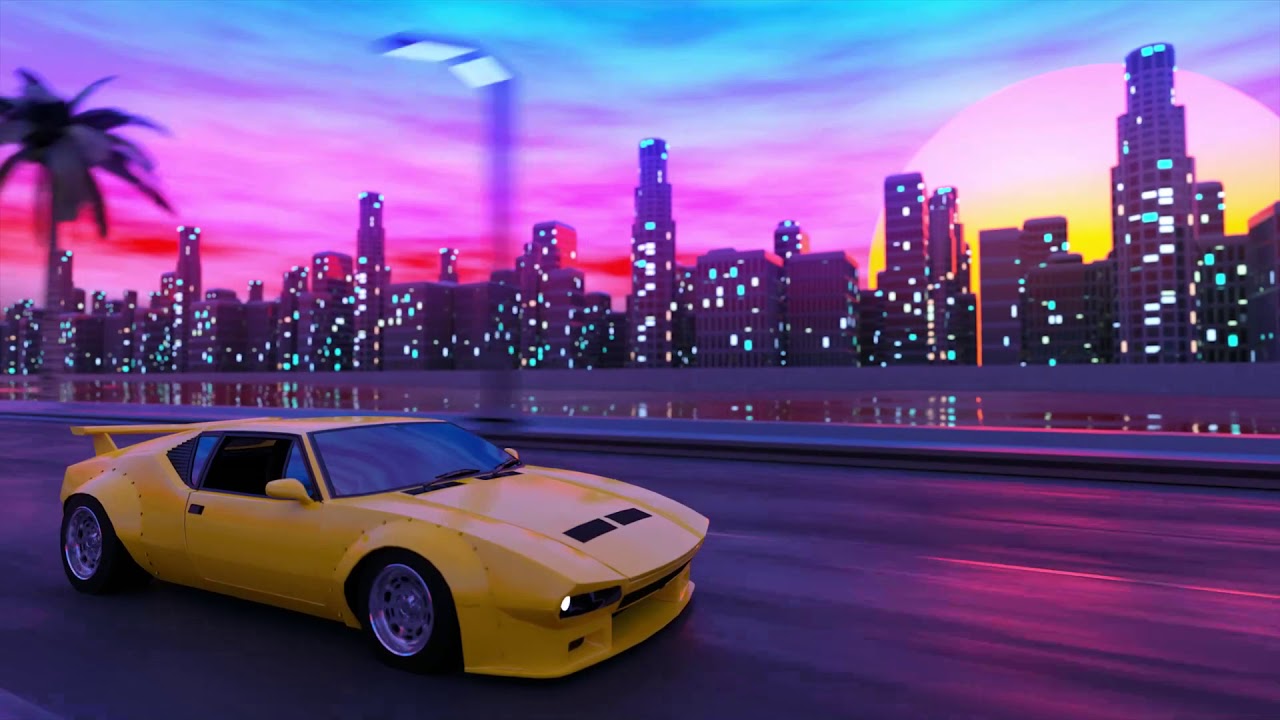
KONFUZ WIKIPEDIA MANUAL
This has necessitated use of machines to understand, interpret and apply data, without manual involvement. The evolution of information Technology has led to the collection of large amount of data, the volume of which has increased to the extent that in last two years the data produced is greater than all the data ever recorded in human history. Since we shall be testing our approach against the existing state of the art as well as entering the area previously not under coverage (Marathi transliterated text), this work is bound to make great strides in the field of sentiment analysis. By this we mean the existing direct supervised learning for sentiment analysis, without much NLP or language specific work. The idea is to not just improve what has already been built or shown to be true, but also check if the simplest approach is still the best way to proceed or not. Although, the current work is a proposal with improvements over established techniques, it is also however going to be quite comparative when it comes to the existing findings. Herein, we assess the existing status, standards and achievements of the researchers in the given field and supplant it without proposed methodology to increase precision. This paper, rather than being a pioneer, is about extending that research for further improvement. This growing research is a result of necessity created through the advent of social media as well as textual analysis of the data being collected online.
KONFUZ WIKIPEDIA CODE
There is a growing research on sentiment analysis of various languages, which is being supplanted heavily by those same techniques and methods being applied on the mix code or transliterated text for the same purpose.
